[Originally posted by Kat on her BacPathGenomics blog, April 2013]
This is a shameless plug for an article and accompanying tutorial I’ve just published together with David Edwards, my excellent MSc Bioinformatics student from the University of Melbourne. It’s currently available as a PDF pre-pub from BMC Microbial Informatics and Experimentation, but the web version will be available soon. The accompanying tutorial is available here.
The idea for this came from discussions at last year’s ASM (Australian Society of Microbiology) meeting, where it was highlighted that there was a lack of courses and tutorials available for biologists to learn the basics of genomic analysis so that they can make use of next gen sequencing. Michael Wise, a founding editor of BMC Microbial Informatics and Experimentation based at UWA in Perth, suggested the new journal would be an ideal home for such a tutorial… so here we are:
http://www.microbialinformaticsj.com/content/3/1/2/
High throughput sequencing is now fast and cheap enough to be considered part of the toolbox for investigating bacteria, and there are thousands of bacterial genome sequences available for comparison in the public domain. Bacterial genome analysis is increasingly being performed by diverse groups in research, clinical and public health labs alike, who are interested in a wide array of topics related to bacterial genetics and evolution. Examples include outbreak analysis and the study of pathogenicity and antimicrobial resistance. In this beginner’s guide, we aim to provide an entry point for individuals with a biology background who want to perform their own bioinformatics analysis of bacterial genome data, to enable them to answer their own research questions. We assume readers will be familiar with genetics and the basic nature of sequence data, but do not assume any computer programming skills. The main topics covered are assembly, ordering of contigs, annotation, genome comparison and extracting common typing information. Each section includes worked examples using publicly available E. coli data and free software tools, all which can be performed on a desktop computer.
Four great tools
In the paper and tutorial, we introduce the four tools which we rely on most for basic analysis of bacterial genome assemblies: Velvet, ACT, Mauve and BRIG. All except ACT were developed as part of a PhD project, and have endured well beyond the original PhD to become well-known bioinformatics tools. New students take note!
In the paper, each tool is highlighted in its own figure, which includes some basic instructions. This is reproduced below, but is covered in much more detail in the tutorial that comes with the paper (link at the bottom).
1. Velvet for genome assembly
Possibly the most popular and widely used short read assembler, developed by the amazing Dan Zerbino during his PhD at EBI in Cambridge. Quite a PhD project!
[ Download | Paper | Protocol ]
Reads are assembled into contigs using Velvet and VelvetOptimiser in two steps, (1) velveth converts reads to k-mers using a hash table, and (2) velvetg assembles overlapping k-mers into contigs via a de Bruijn graph. VelvetOptimiser can be used to automate the optimisation of parameters for velveth and velvetg and generate an optimal assembly. To generate an assembly of E. coli O104:H4 using the command-line toolVelvet:
• Download Velvet [23] (we used version 1.2.08 on Mac OS X, compiled with a maximum k-mer length of 101 bp)
• Download the paired-end Illumina reads for E. coli O104:H4 strain TY-2482 (ENA accession SRR292770)
• Convert the reads to k-mers using this command:
velveth out_data_35 35 -fastq.gz -shortPaired -separate SRR292770_1.fastq.gz SRR292770_2.fastq.gz
• Then, assemble overlapping k-mers into contigs using this command:
velvetg out_data_35 -clean yes -exp_cov 21 -cov_cutoff 2.81 -min_contig_lgth 200
This will produce a set of contigs in multifasta format for further analysis. See Additional file 1: Tutorial for further details, including help with downloading reads and using VelvetOptimiser.
2. ACT for pairwise genome comparison
Part of the Sanger Institute’s Artemis suite of tools. Also look at Artemis (single genome viewer), DNA Plotter (which can draw circular diagrams of your genomes) and BAMView (which can display mapped reads overlaid on a reference genome), they are all available here.
[ Download | Paper | Manual ]
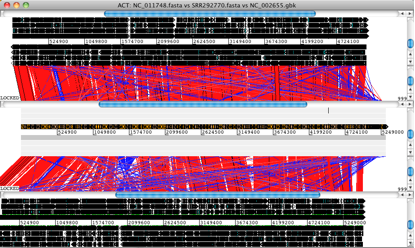
Artemis and ACT are free, interactive genome browsers (we used ACT 11.0.0 on Mac OS X).
• Open the assembled E. coli O104:H4 contigs in Artemis and write out a single, concatenated sequence using File -> Write -> All Bases -> FASTA Format.
• Generate a comparison file between the concatenated contigs and 2 alternative reference genomes using the website WebACT.
• Launch ACT and load in the reference sequences, contigs and comparison files, to get a 3-way comparison like the one shown here.
Here, the E. coli O104:H4 contigs are in the middle row, the enteroaggregative E. coli strain Ec55989 is on top and the enterohaemorrhagic E. coli strain EDL933 is below. Details of the comparison can be viewed by zooming in, to the level of genes or DNA bases.
3. Mauve for contig ordering and multiple genome comparison
Developed by the wonderful Aaron Darling during his PhD, he is now Associate Professor at University of Technology Sydney. Also see Mauve Assembly Metrics, an optional plugin for assessing assembly quality which was developed for the Assemblathon.
[ Download | Paper | User Guide ]
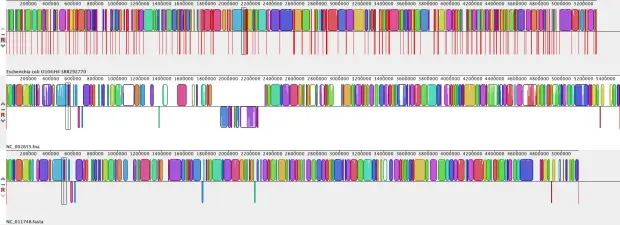
Mauve is a free alignment tool with an interactive browser for visualising results (we used Mauve 2.3.1 on Mac OS X).
• Launch Mauve and select File -> Align with progressiveMauve
• Click ‘Add Sequence…’ to add your genome assembly (e.g. annotated E. coli O104:H4 contigs) and other reference genomes for comparison.
• Specify a file for output, then click ‘Align…’
• When the alignment is finished, a visualization of the genome blocks and their homology will be displayed, as shown here. E. coli O104:H4 is on the top, red lines indicate contig boundaries within the assembly. Sequences outside coloured blocks do not have homologs in the other genomes.
4. BRIG (BLAST Ring Image Generator) for multiple genome comparison
From Nabil-Fareed Alikhan at the University of Queensland, also as part of a graduate project, which I believe is still in progress…
[ Download | Download BLAST | Paper | Tutorial ]

BRIG is a free tool that requires a local installation of BLAST (we used BRIG 0.95 on Mac OS X). The output is a static image.
• Launch BRIG and set the reference sequence (EHEC EDL933 chromosome) and the location of other E. coli sequences for comparison. If you include reference sequences for the Stx2 phage and LEE pathogenicity island, it will be easy to see where these sequences are located.
• Click ‘Next’ and specify the sequence data and colour for each ring to be displayed in comparison to the reference.
• Click ‘Next’ and specify a title for the centre of the image and an output file, then click ‘Submit’ to run BRIG.
• BRIG will create an output file containing a circular image like the one shown here. It is easy to see that the Stx2 phage is present in the EHEC chromosomes (purple) and the outbreak genome (black), but not the EAEC or EPEC chromosomes.
Tutorial
The tutorial accompanying the article is available here. To give you an idea of what’s covered, here is the table of contents:
1. Genome assembly and annotation…………………………………………………………… 2
1.1 Downloading E. coli sequences for assembly…………………………………………….. 2
1.2 Examining quality of reads (FastQC)………………………………………………………… 2
1.3 Velvet – assembling reads into contigs………………………………………………………. 4
1.3.1 Using VelvetOptimiser to optimise de novo assembly with Velvet………….. 6
1.4 Ordering contigs against a reference using Mauve………………………………………. 7
1.4.1 Viewing the ordered contigs (Mauve)………………………………………………… 10
1.4.2 Viewing the ordered contigs (ACT)……………………………………………………. 13
1.5 Mauve Assembly Metrics – Statistical View of the Contigs………………………… 15
1.6 Annotation with RAST……………………………………………………………………………. 15
1.6.1 Alternatives to RAST………………………………………………………………………. 19
2. Comparative genome analysis……………………………………………………………….. 20
2.1 Downloading E. coli genome sequences for comparative analysis………………. 20
2.2 Mauve – for multiple genome alignment……………………………………………………. 21
2.3 ACT – for detailed pairwise genome comparisons……………………………………… 24
2.3.1 Generating comparison files for ACT…………………………………………………. 24
2.3.2 Viewing genome comparisons in ACT……………………………………………….. 27
2.4 BRIG – Visualizing reference-based comparisons of multiple sequences……… 29
3. Typing and specialist tools……………………………………………………………………. 34
3.1 PHAST – for identification of phage sequences…………………………………………. 34
3.2 ResFinder – for identification of resistance gene sequences………………………… 34
3.3 Multilocus sequence typing…………………………………………………………………….. 34
3.4 PATRIC – online genome comparison tool………………………………………………… 34
Recent Comments